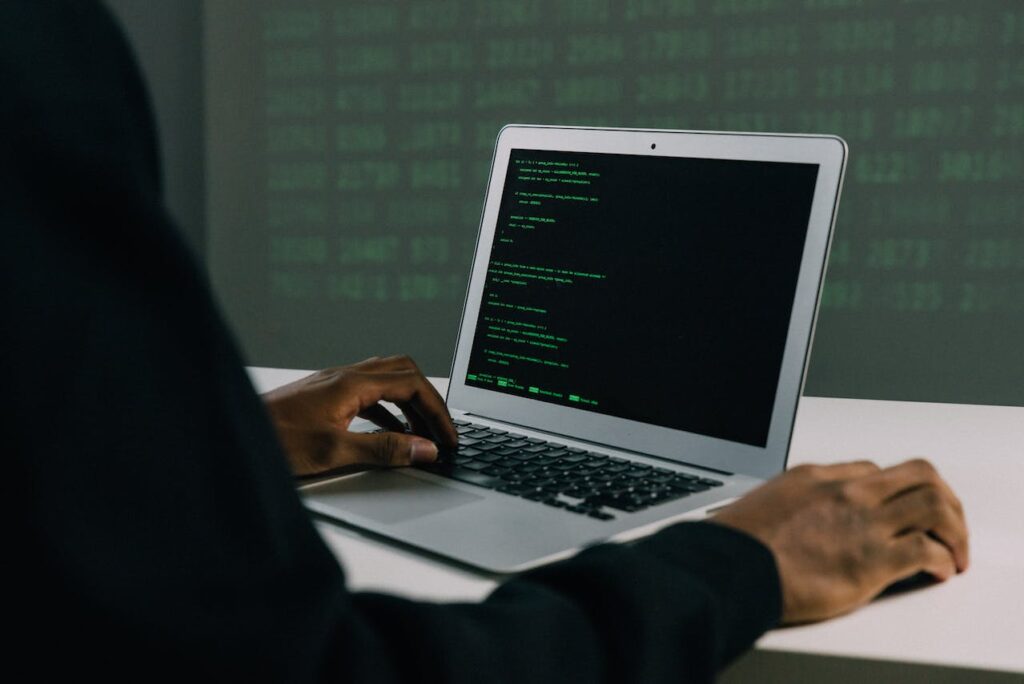
The quality of data, or lack thereof, sets the stage for everything that follows in the development of an AI/ML model. The success of these advanced technologies hinges on the data they are fed. If that data is inconsistent or incomplete, it not only hinders the machine learning process but can also introduce critical errors that compromise the reliability and effectiveness of AI models. This is exactly what necessitates the importance of data enrichment processes when developing AI/ML solutions.
Let’s understand the importance of data enrichment in creating efficient AI/ML training workflows and how it helps in enhancing the quality of data available for model training.
Key components of data enrichment process
Before we get to the importance of data enrichment in creating efficient AI/ML training workflows, let’s understand the main components of data enrichment processes.
- Augmenting contextual information
Data enrichment involves creating variations of existing data by applying various transformations. This technique helps in expanding the diversity of the dataset. It improves model generalization and reduces the risk of overfitting.
- Standardizing and normalizing data
Standardization is done to ensure that data adheres to a consistent format and structure. This step involves converting data into a standardized representation for better compatibility and analysis.
Normalization of data is done by adjusting data values to a common scale or range. It is particularly important when dealing with numerical data to avoid biases caused by disparate value ranges.
- Handling missing data
Data enrichment process includes methods to handle missing values, such as imputation techniques or incorporating external data sources to fill gaps. This ensures that the model is trained on a more complete and representative dataset.
- Feature engineering
Data enrichment often involves creating new features or transforming existing ones to extract more relevant information. Feature engineering is a critical aspect of enhancing the discriminatory power of models as it allows them to capture intricate patterns within the data.
Benefits of data enrichment in AI/ML training workflows
Here are several ways in which data enrichment contributes to the effectiveness of AI/ML training:
I. It forms the foundation of robust AI/ML models
- Quality over quantity
The old adage “garbage in, garbage out” holds particularly true in the domain of AI and ML. The effectiveness of models depends upon the quality of the data used for training. Data enrichment is done to enhance the quality of raw data by filling in the gaps, rectifying errors, and ensuring a consistent format.
- Enhancing feature space
Data enrichment extends beyond simple corrections. It involves the addition of relevant features that contribute to a more comprehensive understanding of the data. For instance, enriching customer data with socio-demographic information or historical behavior patterns can provide valuable context for predicting future actions. This expanded feature space equips AI/ML models with a more nuanced understanding. As a result, it enables them to make more accurate and insightful predictions.
II. It helps tackle the challenges of dealing with raw data
- Incompleteness and missing values
Raw data often arrives with missing values, making it challenging for AI/ML models to discern patterns and relationships accurately. Data enrichment techniques, such as imputation and extrapolation, help fill in these gaps, ensuring a more complete and robust dataset for training.
- Noise and inconsistencies
Noise, in the form of outliers or inconsistencies, can significantly impact the performance of AI/ML models. Data enrichment process involves identifying and rectifying such anomalies, thereby fostering a more reliable dataset. This meticulous cleaning process is crucial for building models that generalize well to real-world scenarios.
III. It assist in tailoring data for specific use cases
- Industry-specific enrichment
Different industries require distinct sets of information for optimal AI/ML model performance. Data enrichment allows organizations to tailor their datasets to industry-specific needs. For example, in healthcare, patient data may be enriched with medical histories and genetic information, while in finance, transactional data may be augmented with economic indicators. This customization ensures that AI models are finely tuned to deliver relevant insights within a given sector.
- Geospatial and temporal enrichment
Certain applications demand an understanding of the geographical and temporal context of the data. Enriching datasets with location-based information or time stamps can be critical for applications such as predicting traffic patterns, optimizing supply chains, or analyzing consumer behavior trends. By considering the spatiotemporal dimension, AI/ML models can offer more contextually aware predictions.
IV. It enables transfer learning and pre-trained models
- Transfer learning
Data enrichment facilitates the implementation of transfer learning, a technique where a model trained on one task is repurposed for another related task. Enriched datasets, with their added contextual information, make it easier for models to transfer knowledge gained from one domain to another. This accelerates the training process and enhances the adaptability of AI/ML models across diverse applications.
- Pre-trained models
The rise of pre-trained models, such as BERT and GPT, further highlights the significance of enriched data. These models, trained on vast and diverse datasets, leverage the power of data enrichment to understand the nuances of language and context. As a result, organizations can fine-tune these pre-trained models with their specific enriched datasets, saving time and computational resources in the training process.
Ethical considerations in data enrichment
- Privacy and consent
While data enrichment offers immense potential, ethical considerations must not be overlooked. Enriching data often involves integrating information from various sources, raising concerns about privacy and consent. Organizations must prioritize transparency and obtain explicit consent when collecting and enriching personal data to maintain trust and compliance with data protection regulations.
- Bias mitigation
Enriched datasets have the potential to perpetuate biases present in the underlying data sources. Organizations must be vigilant in identifying and mitigating bias during the enrichment process. This involves regular audits, diverse representation in training data, and the implementation of fairness-aware algorithms to ensure that AI/ML models do not inadvertently perpetuate or exacerbate societal biases.
On an endnote
Data enrichment stands out as a transformative force that elevates the quality of training data and eventually leads to more accurate and robust models. As organizations continue to harness the power of AI and ML to drive innovation, the implementation of data enrichment practices will only become more pronounced. However, if your organization does not have the resources to conduct data enrichment practices in-house, then you must consider opting for data enrichment services. That way, you will not only get professional assistance but also a cost-efficient solution for accurate AI/ML training workflows.